Artificial night-time light data extraction
If artificial night-time lights (ANTL) are considered means for safety and security, then what is the optimum level of brightness to ensure safety and security without compromising the environment?
About the training
Artificial Night-time Lights (ANTL) have been widely used in social science research as a robust proxy for economic development, the quantification of disaster impacts, electricity consumption, and various other applications. However, employing ANTL in social science research requires a comprehensive understanding of confounding factors, necessitating their individual consideration based on the specific needs of the application. This training aims to provide hands-on experience in both the theory and application of ANTL.
Program objectives
This program will achieve the following outcomes:
- Raise awareness among emerging global leaders about ANTL.
- Share knowledge on ANTL theory, practice, and measurement techniques.
- Train students from the global south to extract, analyse, and visualise ANTL.
- Understand the limitations of satellite imagery in capturing artificial night-time lights.
Use the tabs below to find the information required to participate in this course.
The program will run for three weeks with two two-hour sessions per week.
The first week will be a single two-hour general session with a keynote talk from an expert to provide the context and importance of ANTL. The following two weeks will have technical sessions. In the final session, participants will present their map and briefly discuss their interpretations of it.
Dates
Pre-training session: 11 June 2024
Week 1: 25-26 June 2024 (Introductory keynote and module 1)
Week 2: 2-3 July 2024 (Module 2)
Week 3: 9-10 July 2024 (Modules 3 and 4)
Times
22:30 NZDT (New Zealand), 15:15 NPT (Nepal), 10:30 WAT (Nigeria)
The training program will encompass core concepts related to ANTL data and get skills in extracting the images tailored to relevant research context.
It is designed to equip participants with fundamental skills for accessing a range of ANTL data repositories, utilising advanced software (where possible open source) to map, understand the quality of data, pre-process data, calculate zonal statistics, and analyse the spatial distribution.
The training has 4 major modules, allowing participants to select the modules that align with their preferences. However, we recommend attending all the modules for better understanding.
Recognising that everyone progresses at their own pace, we have established separate breakout rooms to provide additional support from the technical support team.
Pre-training session
This session will make sure all participants start the course with the same level of data knowledge.
Module one
Module 1.1: Introductions and Theory of ANTL
• Understanding ANTL data
• Discuss the sources and confounding factors of ANTL (Spatial, data constraints, satellite sensors)
Module 1.2: Introduce the scope and limits of ANTL dataset
• Temporal resolutions
• Spatial resolutions
Module two
Introduction to Google Earth Engine
• Upload files from computer and catalogue
• Basic commands
• Display data
Part 4: Experiment with maps
Identify hotspots and potential sources emitting ANTL.
Module three
Module 3.1: Using annual data – plotting the ANTL dataset
• Review the projection methodology concept
• Calculate the zonal statistics
• Export data to tabular data
Module 3.2: Using World Bank GDPdata
• Understanding the annual GDP data
• Download and check the dataset
• Export data
Module 3.3: Using ANTL and GDP data
• Merge two datasets
• Plot the scatter plot and conduct a correlation analysis
Module four
Presentations and communication
Students will present their report and discuss their learning. There will also be a Q & A.
Detailed information about each session is included below.
Date | Module | Session | Activities | Facilitator | Objective | |
---|---|---|---|---|---|---|
Week 1 | 25 June 2024 | Introduction and keynote | Part 1: Why and how of the training and session | 5 slides about the objective team, process and expectation (10 minutes) | Sobia Rose | Learn course, process and expectation |
Part 2: Keynote and Q & A | Keynote: Using Night Light data for socio-economic research (1.15 hour) | Professor John Gibson Department of Economics University of Waikato | Keynote | |||
Q&A with expert (25 min) | Chizoba Obianuju Oranu, University Of Nigeria Nsukka | Q & A | ||||
Summary and Thank you (10 minute) | Professor Margaret Chitiga-Mabugu University of Pretoria | Thank you note. | ||||
26 June 2024 | Module 1 | Part 1: Understand the ANTL | Presentation on what is ANTL, how it is captured, and why it is useful in social, economic and environmental terms. | Dipendra Bhattarai | Observe the data and guess the possible sources of nighttime lights. | |
Practical: From the reading materials find out and list the possible sources of nighttime lights. | ||||||
Part 2: Data sources | Potential data sources and confounding factors of ANTL. | Know open access data source and find the data that is useful for your study. | ||||
Week 2 | 2 July 2024 | Module 2 | Upload data and understand | Extract data and understand the format. | Dipendra Bhattarai, Darcy, Bishal, and WiZelle | Learn mapping |
Identify key issues and resolve | Identify key problem or issues in uploading data and resolve it. | |||||
Map your chosen data | Use open-source ANTL data and map it. | |||||
Play with the maps | Identify the hotspots and potential sources emitting ANTL. | |||||
Week 3 | 3 July 2024 | Module 3 | Part 1: Extract the annual ANTL data | Calculate the zonal statistics and export the data to tabular format. | Learn about application |
These reading materials are useful resources to build your knowledge of the content that will be covered during the course.
Journal articles
Bhattarai, D., Lucieer, A., Lovell, H. and Aryal, J. 2023. Remote sensing of night‐time lights and electricity consumption: A systematic literature review and meta‐analysis. Geography Compass. https://doi.org/.10.1111/gec3.12684
Bhattarai, D. and Lucieer, A. 2024. Random forest regression exploring contributing factors to artificial night-time lights observed in VIIRS satellite imagery. International Journal of Digital Earth, 17. https://doi.org/.10.1080/17538947.2024.2324941
Dingel, J. I., Miscio, A. and Davis, D. R. 2021. Cities, lights, and skills in developing economies. Journal of Urban Economics, 125. https://doi.org/.10.1016/j.jue.2019.05.005
Baragwanath, K., Goldblatt, R., Hanson, G. and Khandelwal, A. K. 2021. Detecting urban markets with satellite imagery: An application to India. Journal of Urban Economics, 125. https://doi.org/.10.1016/j.jue.2019.05.004
Monroe, T. Big Data and Thriving Cities. https://elibrary.worldbank.org/doi/abs/10.1596/26299
Blog
Min, B., Baugh, K., Monroe, T., Goldblatt, R., Stewart, B., Kosmidou-Bradley, W. & Crull, C. (2021). Light every night: New night-time light data set and tools for development. World Bank Blogs.
Useable dataset and tutorial for further information
World Bank. (n.d.). Light every night: Registry of open data on AWST
Training resources are available on the CIET GitHub.
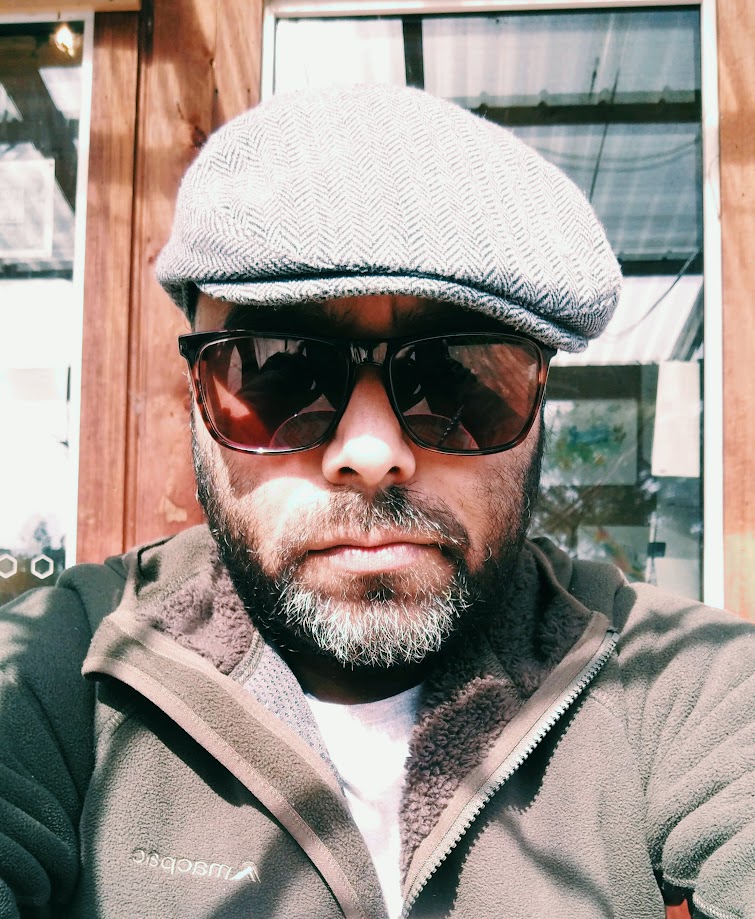
Dipendra Bhattarai
University of Tasmania
Technical lead
Dipendra Bhattarai brings a decade of experience collaborating with grassroots organisations and leveraging remote sensing skills to address the challenges of sustainable development goals in data-scarce regions. His research focuses on artificial night-time lights, energy, and climate policy, utilising data to translate complex information into actionable insights through a blend of economic and spatial rigour.
For more training related information, reach out at dipendra.bhattarai@utas.edu.au or @bdipen on X (Formerly Twitter)
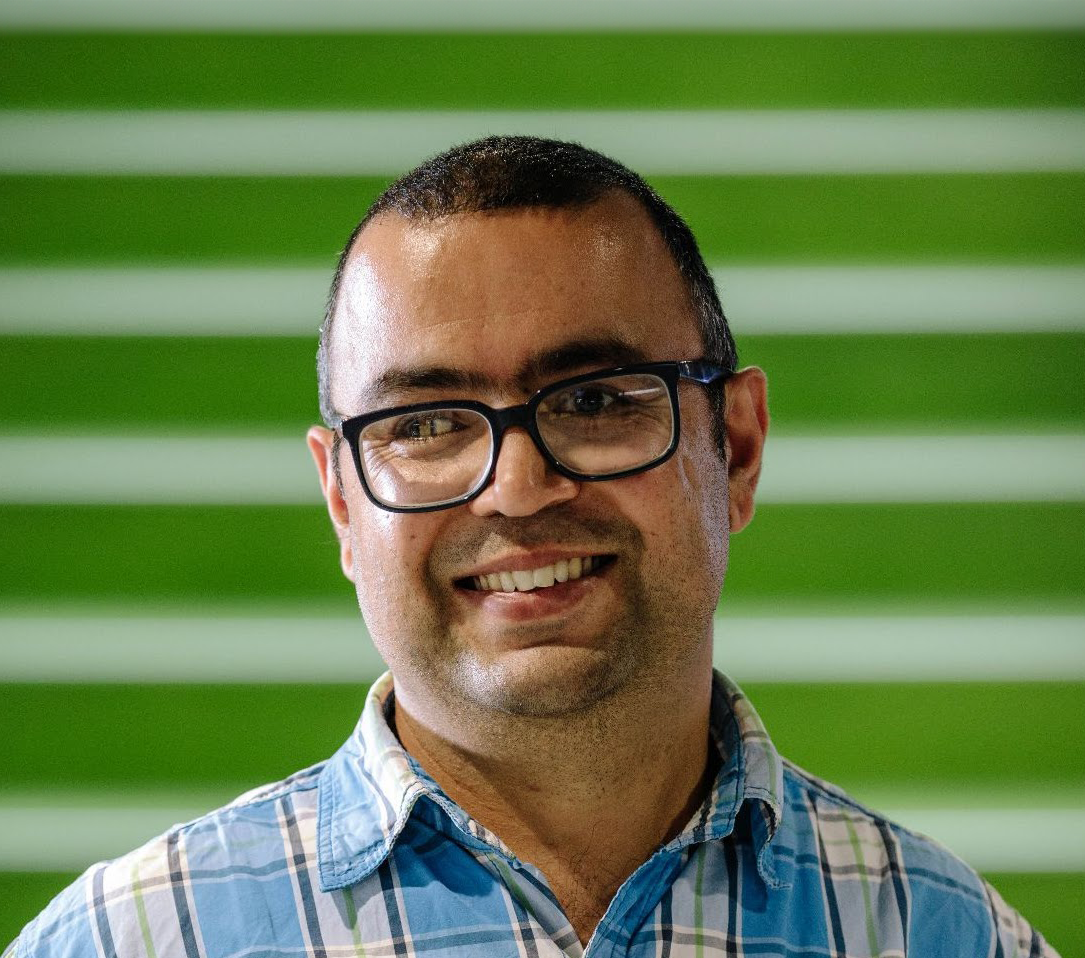
Dr Bishal Bharadwaj
Curtin University, Australia
Training Coordinator
Bishal Bharadwaj is a quantitative economic geographer interested in evaluating environmental policies (energy, plastic, pollution and climate change) in heterogeneous contexts to identify strategies to ensure policies are effective and equitable. He is currently a Research Fellow at the CIET.
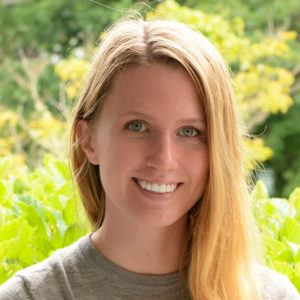
Darcy Glenn
University of Canterbury, New Zealand
Darcy Glenn has worked to bring climate science to local governments so they can make informed decisions. She was a research assistant on Woodwell Climate Research Centre’s Risk team, working on free climate risk assessments for local governments.
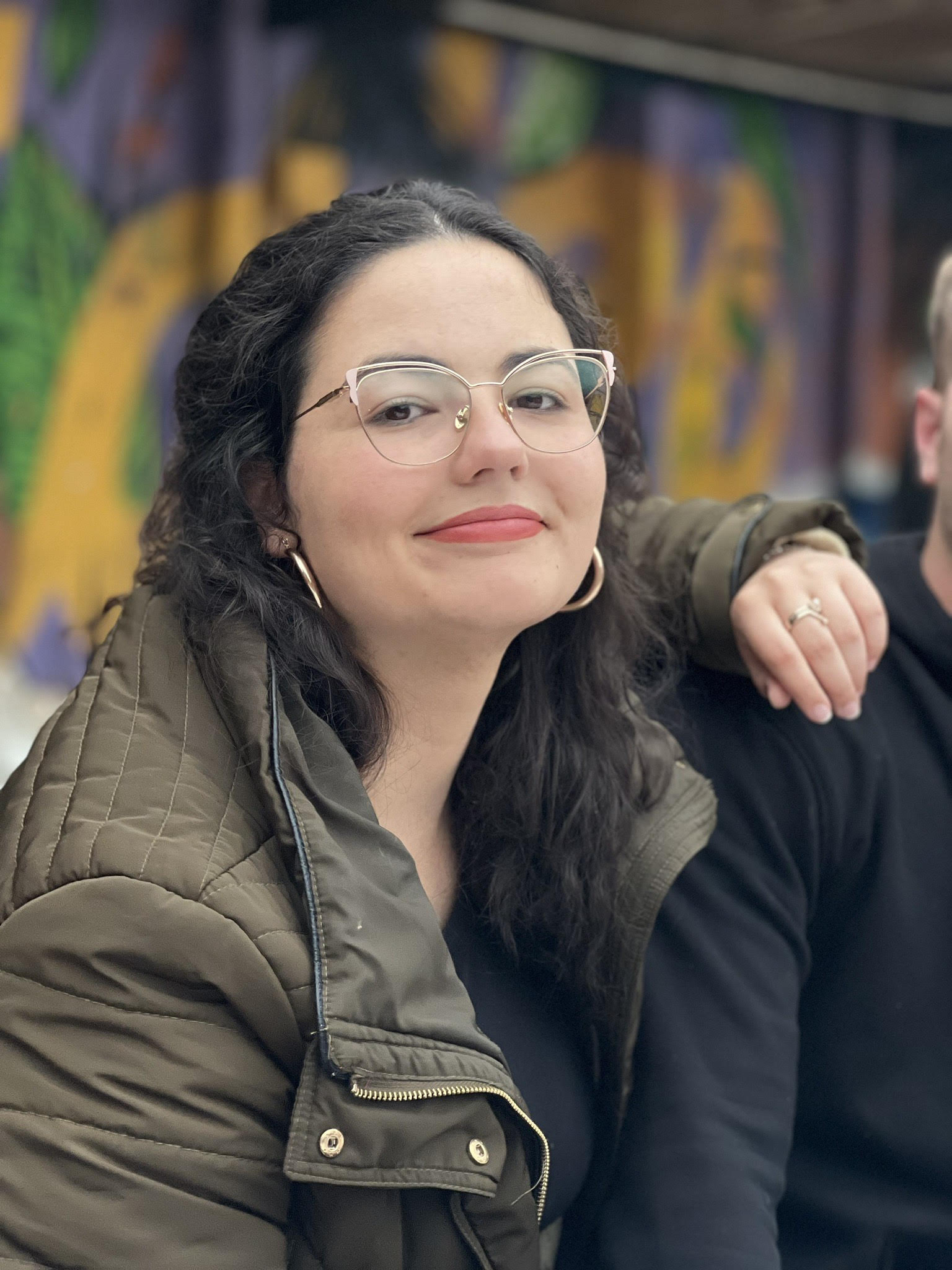
WiZelle Kritzinger
University of Pretoria, South Africa
WiZelle Kritzinger is a Master of Philosophy in Economics student at the University of Pretoria. She is interested in energy and environmental economics, with a keen focus on energy poverty, its causes and consequences. She is currently a research assistant for the University of Pretoria’s Economic and Management Sciences Faculty Funded Transdisciplinary Project on Energy Poverty.
Contact details
If you are registered for this training and need assistance, contact the training or technical coordinators as below. For country-specific questions, reach out to your local coordinator (check the working group for their contact details).